DLP: towards active defense against backdoor attacks with decoupled learning process
Deep learning models are well known to be susceptible to backdoor attack, where the attacker only needs to provide a tampered dataset on which the triggers are injected. Models trained on the dataset will passively implant the backdoor, and triggers on the input can mislead the models during testing. Our study shows that the model shows different learning behaviors in clean and poisoned subsets during training.
For more information: DLP: towards active defense against backdoor attacks with decoupled learning process | Cybersecurity | Full Text (springeropen.com)
Authors: Zonghao Ying & Bin Wu
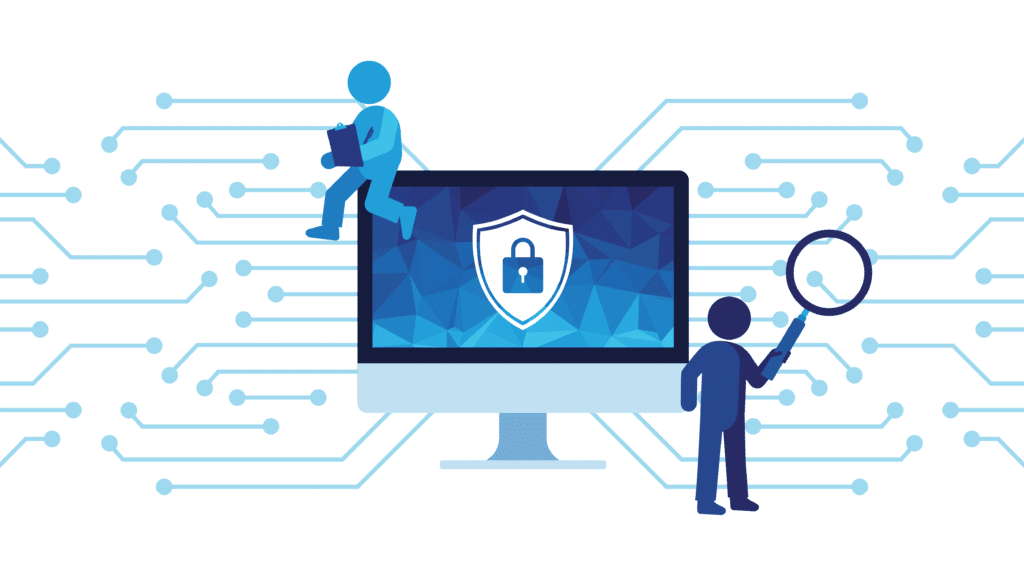